The Age of Digital Twins¶
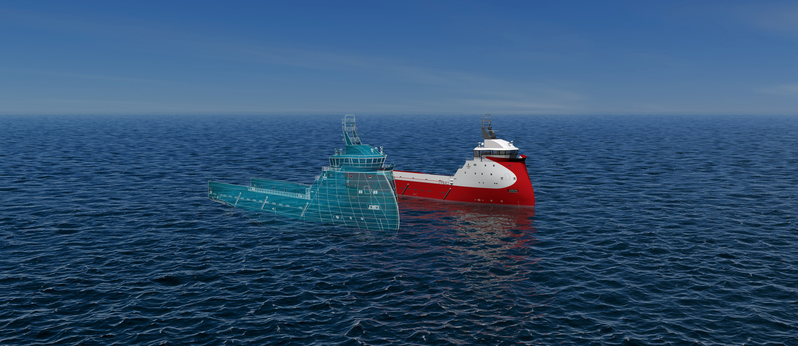
“Digital twinning” was identified by Gartner as one of the top 10 technology trends of 2017 [1]. A digital twin is a digital representation of a physical object, asset, or system, e.g. a ship, a car, a wind turbine, a power grid, a pipeline, or a piece of equipment such as a thruster or an engine. It may contain a collection of various digital models and information related to this object, including 3D models, structural models, process models, ontologies, sensor data records, documentation and reports. Ideally, all quantities and attributes you could measure or observe from the real asset should be accessible from its digital twin.
The novelty of digital twins is not the existence and use of models of assets, but the way in which these models are bundled, linked to data streams and maintained over the assets’ lifetime: A digital twin is not just a model of a type of asset, but a model of a particular asset instance. For example, a digital twin will reflect a unique operating history, and, correspondingly, a unique amount of accumulated “damage”. With a digital twin, decisions about that particular asset can be informed in a very specific way, enhancing both performance and safety. A digital twin reflecting an asset’s condition may, for example, permit more optimal inspection and maintenance scheduling, reducing downtime and unnecessary interventions. A digital twin may also be used to test the asset’s performance in various scenarios given its current condition, thereby avoiding dangerous situations that could compromise the real asset.
The implementation of digital twins is gaining momentum with the rapid evolution and improved affordability of sensor technology, computing power, internet-of-things and data analytics methods. In addition, virtual reality and augmented reality technologies have opened up new possibilities for how decision makers can interact with digital twins and take advantage of the information harboured within them.
Digital twins - definition and elements¶
The idea of digital twins is often attributed to Grieves, who presented the concept in 2002 in the context of Product Life-cycle Management [2]. The idea was that when designing and manufacturing a physical system, a virtual copy should be produced and live alongside it, mirroring the physical system throughout the life-cycle. Grieves and Vickers proposed the following definition of a digital twin [2]:
“The Digital Twin is a set of virtual information constructs that fully describes a potential or actual physical manufactured product from the micro atomic level to the macro geometrical level. At its optimum, any information that could be obtained from inspecting a physical manufactured product can be obtained from its Digital Twin.”
Grieves and Vickers further introduce a taxonomy of digital twins [2], paraphrased below:
- Digital Twin Prototype (DTP): Contains the informational sets necessary to describe and produce a physical version. A DTP is not linked to a particular existing physical asset.
- Digital Twin Instance (DTI): Linked to a specific physical asset, containing information about that asset and its history, captured from sensors, tests, inspections and so on.
- Digital Twin Aggregate (DTA): A computing construct that has access to all DTIs, i.e. an aggregate of a collection of DTIs.
- Digital Twin Environment (DTE): An integrated, multi-domain physics application space for operating on Digital Twins., i.e. interrogation of DTIs for current and past states, and prediction of the future behaviour and performance of physical products under various scenarios using their DTI (and possibly DTA).
Cabos and Rostock [3] propose that a digital twin is constituted by three basic components, which can be described in general terms as follows:
- A digital representation of the object, which relate to the system structure. Such representations could have a visual user interface, e.g. in the form of 3D models, or may consist of system specifications or ontologies describing the system architecture, including components and their interfaces.
- Behavioural models, which describe the dynamic response of the system (i.e. its state and its outputs). Such models may include the operation of the system (i.e. for simulating how the system performs it’s intended functions), the structural response to the loads it is exposed to, as well as degradation and damage processes.
- The configuration or condition of the system, is data records of the past and current state of, or changes to, the system, captured through sensors, inspections etc.
In short, a digital twin is constituted by data and models. Fig. 1 illustrates the possible constituents of a digital twin in more detail.
Note that many real assets are cyber-physical systems, containing both physical (hardware) and cyber (software) elements. While the models contained in a digital twin for the physical elements are approximations of reality, the cyber elements, such as control systems, are already digital and can be included in the digital twin as they are and do not need to be emulated.
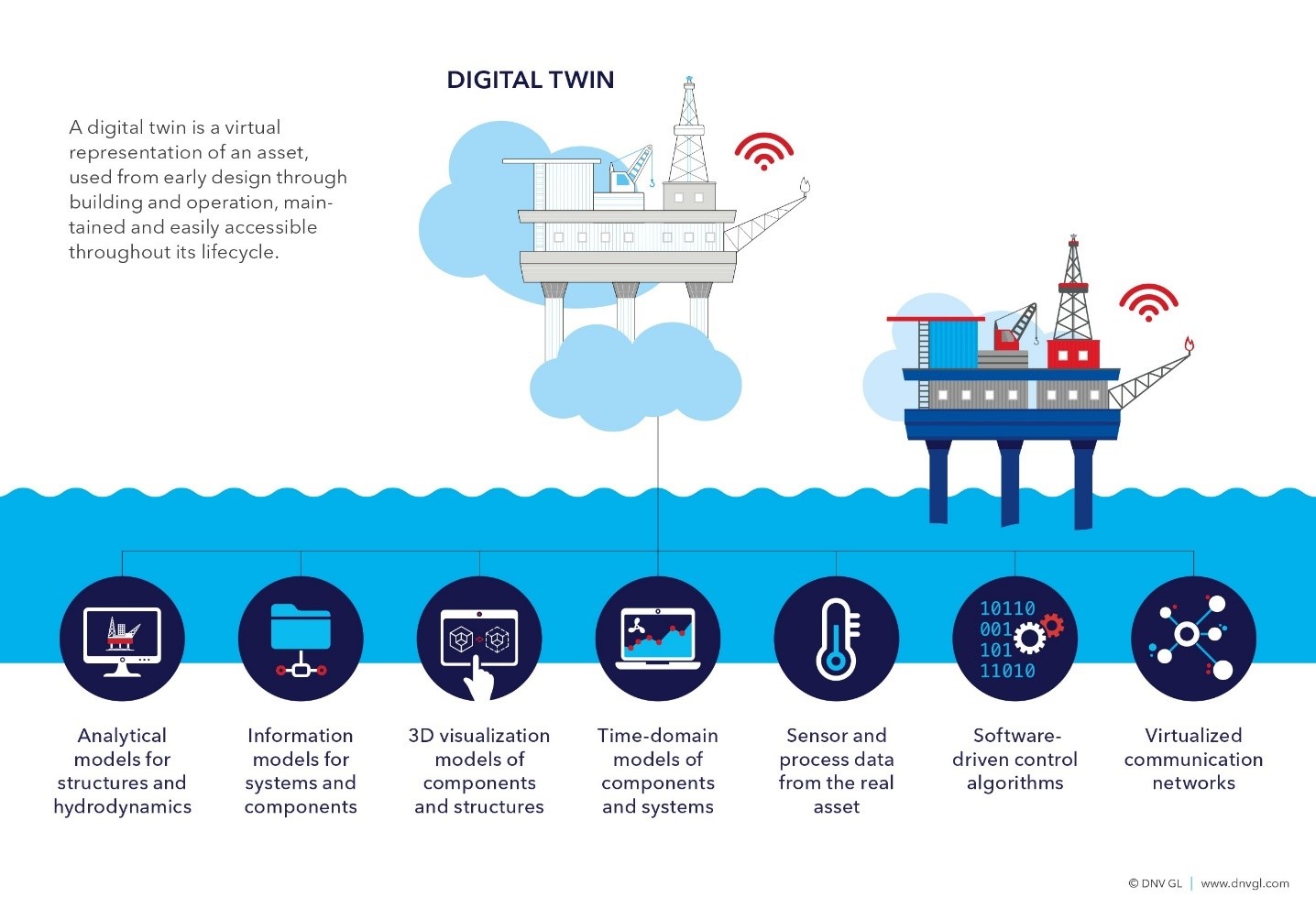
Fig. 1 Illustration of DNV’s digital twin concept for an oil and gas facility.
Uses of digital twins¶
Digital twins may be used in all life-cycles of an asset:
- In the design phase, digital twins can be used to explore un-built assets in virtual reality. They can also be used for virtual system testing, i.e. to explore relevant scenarios and responses prior to building or deploying a physical asset, or when tests or experiments on the real asset would be costly, dangerous or time consuming to perform. Digital twins can also be used for testing and integration of software systems, e.g. by letting the control system control a digital twin instead of the real asset.
- During the manufacturing phase, production processes can be simulated and the product can be monitored and compared to the virtual design version. As Grieves and Vickers say [2], “We can collect the data from the machines that perform operations on the physical part to understand exactly what operations, at what speeds and forces, were applied. For example, we can collect the torque readings of every bolt that attaches a fuel pump to an engine in order to ensure that each engine/fuel pump attachment is successfully performed.”
- In operation, a digital twin may be continuously updated to reflect the current condition of the asset, based on sensor data, test and inspection results, conducted maintenance, modifications etc. Thus, the digital twin can serve as a repository for easy retrieval of information during operations. Remote workers may be able to move around the digital twin in virtual reality, easing collaboration and communication with personnel on site. Similarly, workers on site may be able to retrieve information from the digital twin as they move about on the real asset wearing augmented reality technology. Beyond information retrieval, digital twins can also be used to predict future performance, both by allowing simulation of the asset conditional on its current state and forecasts of its environment, and by extracting insights from the gathered experience data. Digital twins may allow complex and dangerous operations to be simulated before they are carried out in real life, thereby reducing the risk of complications or accidents.
- In the decommissioning phase, after the physical asset is retired, the digital twin can be retained in a “digital graveyard”, providing learning for future projects. Accumulated data from digital twins of retired assets may also help development of improved designs and better predictive models for new or similar assets.
DNV has long been a proponent of digital twins in the industries we serve, and is already building and using digital twins in our services. For example, in the maritime, oil and gas, and energy sectors, we are performing hardware-in-the-loop testing, where digital twins are integrated with real control system to test the control algorithms. We also use digital twins for virtual system integration test, and to simulate virtual experiments instead of performing expensive, destructive or dangerous experiments in real-life. In 2016, DNV developed the NauticusTwinity concept of a virtual sister ship for the maritime industry, for design, testing and verification throughout a vessel’s life cycle [4]. More recently, DNV has joined forces with other industry actors to form a joint open simulation platform, enabling easier reuse and sharing of digital twin models [5]. In the renewable energy sector, DNV develops digital twins of wind farms [6] and solar farms, which supports customers in design and operation. We also offer a digital twin solution for fish farms [7]. More about DNV’s work on digital twins can be found here [8].
To focus on opportunities for using digital twins in risk management, the next section elaborates on the concept of risk, and its dynamic nature. Subsequently, we introduce the probabilistic digital twin as a way to connect digital twins to risk models.
References¶
[1] | Gartner’s Top 10 Strategic Technology Trends for 2017. link |
[2] |
(1, 2, 3, 4)
|
[3] |
|
[4] | K.B. Ludvigsen, L.K. Jamt, N. Husteli and Ø. Smogeli (2016), Digital twins for design, testing and verification throughout a vessel’s life cycle, COMPIT conference, Lecce, Italy. link |
[5] | Creating an Open Source Simulation Platform for the development of new ships. link |
[6] | DNV Windfarmer, link |
[7] | Nautical Site Information Models (NSIM), link |
[8] | Digital Twins at Work in Maritime and Energy, link |