Introduction¶
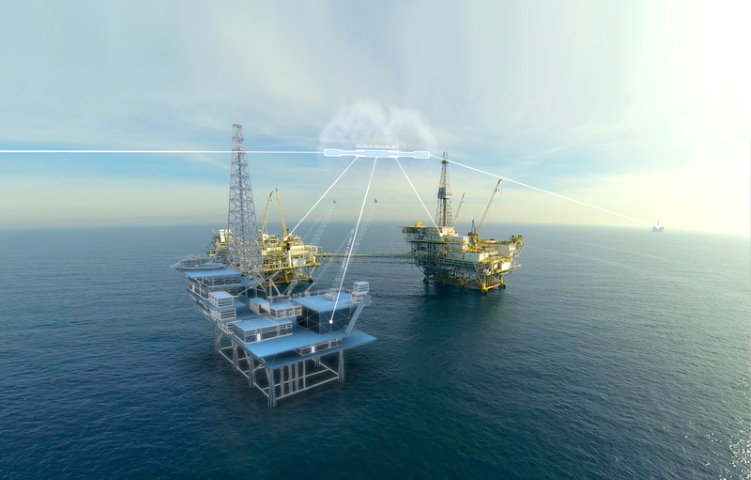
To inform decisions about safety and business strategy, risk assessments have usually been conducted before key decision gates, based on generic historical data and off-line simulations. Meanwhile, we see digital twins emerging all around us; virtual copies of specific physical assets, simulating them in real time and maintaining detailed records of their history. However, digital twins live separately from risk models in most organizations, and the information contained within them is not fully exploited for risk management. Furthermore, digital twins typically do not capture the uncertainties that constitute risks.
By connecting digital twins to dynamic risk models that capture uncertainty about the near-term future, we can provide live risk assessments that reflects the actual condition and operation of the asset
This is the probabilistic digital twin (PDT).
This position paper describes the concept of PDTs and demonstrates it through an example.
Key positions¶
1) Current risk models used in safety management often lack real-time capabilities.
Risk models used in design to inform long-term decisions take into account all that can happen during a system’s lifetime, and often present risk in terms of time averages. However, conditions that are uncertain in design or that vary over time may be known or fixed in operation, and typically vary less in the short term than in the long term.
To be useful for managing safety in operation, risk models should capture dynamics on the time-scale relevant for decisions and take into account new knowledge and changes in conditions that affect the risk level.
2) Digital twins typically miss the uncertainties that matter when it comes to risk.
Digital twins record an asset’s history and contain model for simulating how it works, while risk assessment are concerned with the asset’s future condition and simulating how it can fail. Although the digital twins contain a lot of asset-specific information that can be used to support more detailed and updated risk assessments, the information contained in a digital twin may also be incomplete and imperfect. This uncertainty is important! Risk is not only about what we expect to happen, but also about less likely events that may carry large consequences.
Moreover, computationally heavy high-fidelity models contained in digital twins may not be suitable for use in risk assessments, due to the need to propagate uncertainty. This can increase computational costs by additional orders of magnitude, and thus may not deliver decision support in the relevant time-frame.
3) A probabilistic risk model layer on top of digital twins can enable live risk assessments based on actual conditions, forecasts and plans.
By adding a probabilistic layer to digital twins, uncertainty may be expressed and updated based on varying conditions and new knowledge. The probabilistic layer may include probabilistic failure and degradation models, probabilistic logic and relational models to model scenarios, uncertain relationships, and probabilistic surrogate models (i.e. fast approximations) to more heavy simulation models in the digital twin.
4) Probabilistic digital twins allow operators to manage safety and production more optimally throughout the life-cycle of the asset.
PDTs highlight how operational conditions, the status of safety barriers, and so on, influence risk in real-time. It allows operators to explore the effect of, and identify, operational strategies to avoid or compensate for conditions or events that increase the risk. PDTs support operational decisions related to maintenance and inspection planning, task scheduling, safe job analysis and so on. Making digital twins probabilistic from the concept and design phase enables new capabilities for virtual testing, assurance and certification.
Probabilistic digital twins fill the gap between digital twins and risk management.